The WholeTraveler Transportation Behavior Study explores the energy implications of behavioral factors associated with the adoption and use of emerging transportation technologies and services, including connected and automated vehicles, mobility-on-demand, electric vehicles, and e-commerce.
Berkeley Lab researchers analyze behavioral factors, including underlying barriers to adoption, drivers of adoption, and use of these emerging technologies and more traditional transportation modes — such as personal vehicles, public transit, and walking/biking — informed by the travelers’ life contexts.
The project uses an innovative, regionally focused survey designed to reveal the relationships between pivotal population characteristics, attitudes, and preferences. The survey data, available through the Livewire Data Platform (https://livewire.energy.gov/), will indicate how people will likely use those technologies and services, the resulting impacts on the transportation system, and implications for energy. The WholeTraveler team tackled several research topics.
Life-Course Patterns and Mobility Choices
This project considers how individuals’ phases in life, and the patterns in which they progress through those phases, impact their mobility behaviors and decisions. Researchers identified focused on these key phases: being in school, being employed, living with a partner, and having children. The study applied machine learning techniques to classify survey respondents into five patterns characterizing how they progress through these key phases.
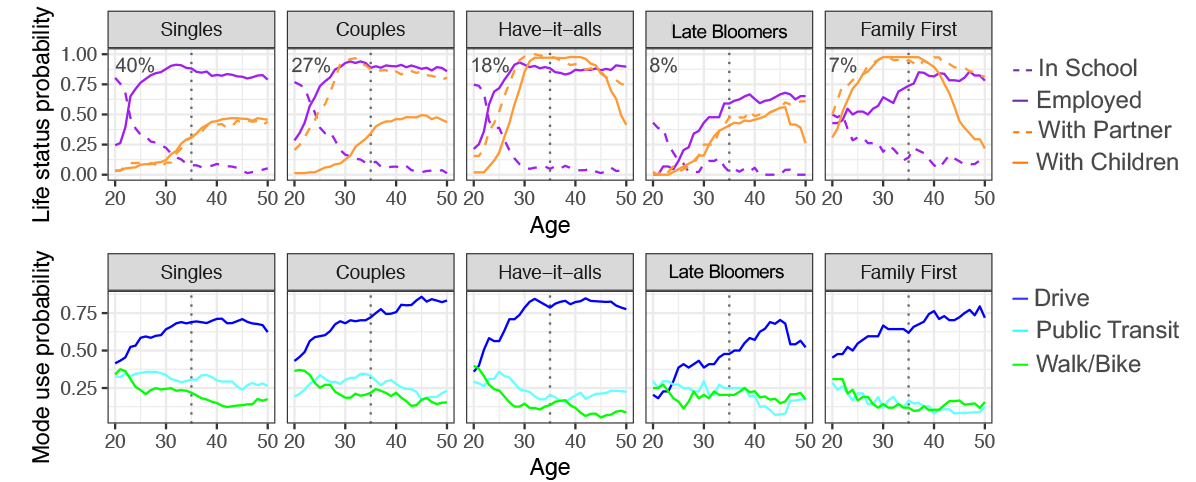
These patterns were related to their concurrent mobility choices to generate insights regarding the underlying forces influencing their mobility behaviors. The study found that the timing and order of key life events can impact mobility choices and that events happening relatively earlier in life have more significant effects. For example, the study found that having children can drive a faster transition to more vehicle-dependence for some people, but not necessarily for others.
The following set of publications describe results from this research effort:
- Jin, Ling, Alina Lazar, James Sears, Annika Todd, Alex Sim, Kesheng Wu, Hung-Chia Yang, and C. Anna Spurlock. "Clustering Life Course to Understand the Heterogeneous Effects of Life Events, Gender, and Generation on Habitual Travel Modes." IEEE Access (2020) 1-17. eta.lbl.gov/publications/clustering-life-course-understand
- Lazar, Alina, Alexandra Ballow, Ling Jin, C. Anna Spurlock, Alex Sim, and Kesheng Wu. "Machine Learning for Prediction of Mid to Long Term Habitual Transportation Mode Use." 2019 IEEE International Conference on Big Data (Big Data)2019 IEEE International Conference on Big Data (Big Data). Los Angeles, CA, USA: IEEE, 2019. eta.lbl.gov/publications/machine-learning-prediction-mid-long
The following set of publications describe work on the methodology used in this research effort:
- Lazar, Alina, Ling Jin, C. Anna Spurlock, Kesheng Wu, Alex Sim, and Annika Todd. "Evaluating the Effects of Missing Values and Mixed Data Types on Social Sequence Clustering Using t-SNE Visualization." Journal of Data and Information Quality 11.2 (2019) 1 - 22. eta.lbl.gov/publications/evaluating-effects-missing-values
- Lazar, Alina, Ling Jin, C. Anna Spurlock, Annika Todd, Kesheng Wu, and Alex Sim. "Data quality challenges with missing values and mixed types in joint sequence analysis." 2017 IEEE International Conference on Big Data (Big Data)2017 IEEE International Conference on Big Data (Big Data). Boston, MA, USA: IEEE, 2017. eta.lbl.gov/publications/data-quality-challenges-missing
Describing the Users: Understanding Adoption of and Interest in Shared, Electrified, and Automated Transportation
Another study identified traits that indicate likely adoption of technology. Berkeley Lab researchers correlated individual and household characteristics with interest in and adoption of multiple emerging technologies and services.
They leveraged data from a novel survey of San Francisco Bay Area residents to analyze adoption patterns for shared mobility, electrified vehicle technologies, and vehicle automation. They found that ride-hailing and adaptive cruise control have penetrated the market more extensively than have electrified vehicles or carsharing services. Over half of respondents have adopted or expressed interest in adopting all levels of vehicle automation. Overall, there is substantial potential for market growth for the technologies and services they analyzed.
Using county fixed effects regressions, they investigated which individual and location-level factors correlate to adoption and interest. They found that, although higher-income people are disproportionately represented among current adopters of most new technologies and services, low- to middle-income people are just as likely to have adopted pooled ride-hailing.
Younger generations have high interest in automated and electrified vehicles relative to their current adoption of these technologies, suggesting that young people could contribute substantially to future market growth — as they are doing for ride-hailing. They found no evidence that longer commutes present a barrier to plug-in electric vehicle adoption.
Finally, women are less likely than men to adopt and/or be interested in adopting most new transportation technologies, with the exception of ride-hailing. There was a significant gender gap in adopting plug-in electric vehicles. A mediation analysis demonstrated that over 20% of the gender gap could be explained by women’s mobility needs and the lower household income of female survey respondents compared to male participants. Specifically, the study found women need to make multiple stops, need more cargo and seating space, and need to transport children. Designing or marketing technologies with women’s preferences in mind could contribute to future market expansion.
See a preprint of the journal article that summarizes this research, "Describing the users: Understanding adoption of and interest in shared, electrified, and automated transportation in the San Francisco Bay Area." Transportation Research Part D: Transport and Environment 71 (2019) 283-301.
Impact of Home Delivery on Shopping Trips
Berkeley Lab researchers conducted an in-depth analysis of e-commerce behavior and the degree to which receiving home delivery of items replaced or added to household shopping trips. The study, based on survey data collected in 2018, found a given delivery was 1.7 times as likely to replace a shopping trip as not, and 1.3 times as likely to replace a vehicle shopping trip than add on to existing trips.
For more information, please see the following journal article: "Children, Income and the Impact of Home Delivery on Household Shopping Trips."
The WholeTraveler Transportation Behavior Study is a pivotal project of the Mobility Decision Science pillar of the Systems and Modeling for Accelerated Research in Transportation (SMART) Mobility Consortium, funded by the Energy Efficient Mobility Systems (EEMS) program of the U.S. Department of Energy (DOE), Vehicle Technologies Office.